Do you have a SAFTE-FAST project file open right now? If the answer is yes, please do me a favor and check the toolbar. Do you see the icon that looks like a dartboard with an arrow hitting the center? That is the Insights icon. A dead giveaway is that the toolbar says “Insights” under the icon.
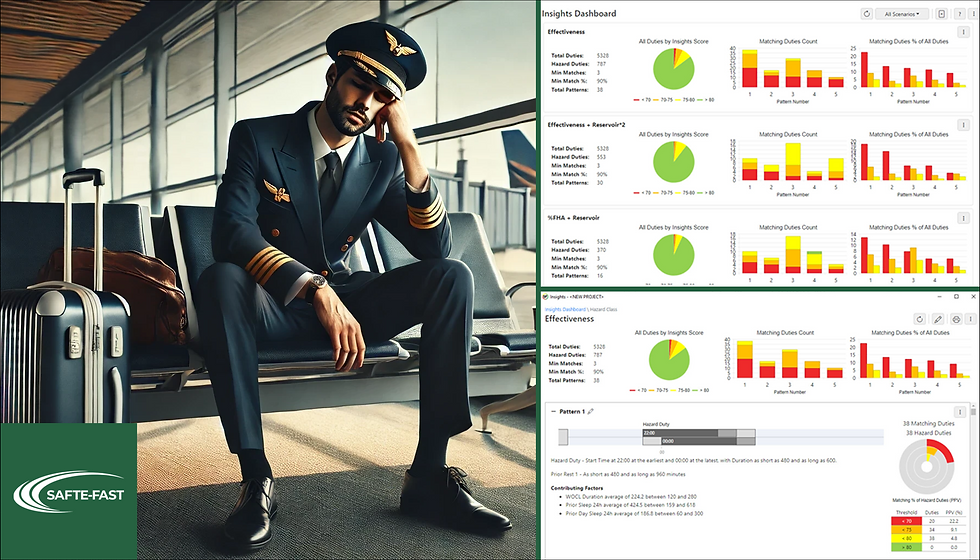
Insights is a recently added analytical tool in SAFTE-FAST that looks for patterns of schedule features that consistently result in high fatigue predictions. Insights scans all schedules in a project file to identify duties that meet user-selected criteria such as low Effectiveness, low Sleep Reservoir, and/or high Workload to evaluate whether features such as early starts, long duty periods or prior rest consistently contribute to fatigue risk. Insights does this using a method called signal detection theory[1]. Signal detection theory is a framework for distinguishing between information-rich patterns and random noise. The process is similar to machine learning. In SAFTE-FAST, Insights is searching for combinations of work events that are leading to fatigue.
For example, let’s say an operator worked a 5-hour overnight shift. Then, they had a day off but needed to return to work early the following day for a 10-hour long day shift. By the end of that day shift, SAFTE-FAST would show that the worker is exhausted due to lack of prior sleep and circadian disruption. However, the day shift isn’t the problem; the combination of a long day shift with an early start time that followed an overnight shift is the problem.
Insights is designed to pick up on a fatiguing pattern, but how do we know that the analytical tool is accurate? One way to check is to compare SAFTE-FAST predictions against real world incidences of fatigue. In aviation, we can test the accuracy of Insights predictions against incidences of fatigue reporting. Crewmembers are encouraged to submit voluntary fatigue reports when they feel that they cannot complete their duty as scheduled due to fatigue, in a process that is similar to “calling in sick”. Reports generally include the time of the occurrence and the flight details in addition to the information about fatigue. Using this information, the report can be linked back to the roster. Fatigue reports are underutilized as a real-world measure of fatigue risk that can be used as a means to evaluate the accuracy of predictive modeling.
A major United States-based airline recently shared one month’s worth of planned and completed rosters for their narrow-body fleet and the fatigue reports associated with each roster with the IBR science team. The IBR team tested the ability of Insights to predict which rosters had duties that resulted in the submission of a fatigue report based on model estimates of alertness, sleep debt, and workload using an odds ratio analysis. Odds ratio is a statistical test to quantify the strength of the association between two events. In this case, the first event was whether a duty was identified as high fatigue risk by Insights—we call this a “hit”-- and the second event was whether a fatigue report had been submitted for that same duty.
Insights could correctly identify a planned duty with a fatigue report over two times more frequently than random chance alone. Conversely, Insights was actually less likely than random chance to identify a duty with fatigue risk for completed duties. These findings make sense because the submission of the fatigue report likely resulted in a change to the roster that would reduce risk in real time. Comparing the planned rosters to the completed rosters revealed that the completed duties started later in the day and were shorter in duration than planned duties. Planned duties that later resulted in a fatigue report were scheduled to start earlier in the day than duties that did not result in a fatigue report.
In short, what the odds ratio found was that Insights does a good job of prospectively identifying fatigue risk, as it did for the planned rosters, and furthermore does not identify risk in similar duties where changes have been made to mitigate fatigue, like what happened for the completed rosters. The full report of this analysis is currently under peer review and should be published in an academic journal soon. For more blog posts about Insights, check out Insights Into What Is Driving Fatigue In Your Schedule Construction and Stop the Insanity of Mitigating the Same Fatigue Month after Month. Also, feel free to click the Insights icon if you are curious about using the tool for your own analyses.
References
Comments